碧桂园政经名家大讲堂——陈伟坤博士学术报告
报告题目一:Exact separation algorithm for unsplittable capacitated network design flow arc-set polyhedron
报告时间:2019年12月19日(星期四)上午9:30-11:00
报告地点:中国西部科技创新港5号楼(经济金融研究院)5-8088
报告摘要:
Many practical problems arising in real world applications, mainly in the fields of logistics and telecommunications, can be modeled as unsplittable capacitated network design problem. In this talk, we consider the flow arc-set polyhedron of this problem. By analyzing the property of its nontrivial facet, we design an exact algorithm to solve the associated separation problem such that the constructed inequality guarantees to be facet-defining. To speed up this approach, we present a closed form of the separation problem under some conditions. Furthermore, a new technique is presented to accelerate the exact separation algorithm, which significantly decreases the number of iterations in the algorithm. Finally, a comprehensive computational study on the unsplittable capacitated network design problem is presented to demonstrate the effectiveness of the proposed algorithm.
报告人简介:
陈伟坤,北京理工大学特聘副研究员。2014年本科毕业于中山大学数学与计算科学学院,2019年在中国科学院数学与系统科学研究院获得博士学位。博士期间,受中国科学院大学资助访问德国Zuse Institute Berlin一年(合作导师:Thorsten Koch教授)。毕业后,他就职于北京理工大学数学与统计学院。他的主要研究兴趣是整数规划算法与应用,相关的研究成果发表在《IEEE Journal on Selected Areas in Communications》,《Journal of Global Optimization》,《Science China Mathematics》等期刊上。2018年获得中国运筹学会颁发的“科学技术奖运筹应用奖”,2019年获得中国数学会计算数学分会颁发的优秀青年论文二等奖。
报告题目二:Stochastic polynomial optimization
报告时间:2019年12月23日(星期一)下午15:00-17:00
报告地点:西安交大财经校区教学楼612
报告摘要:
This talk is about stochastic optimization problems with polynomials. We propose an optimization model with sample averages and perturbations. The Lasserre type Moment-SOS relaxations are used to solve the sample average optimization. Properties of the optimization and its relaxations are studied. Numerical experiments are presented.
报告人简介:
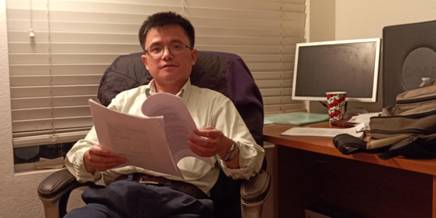
聂家旺,现为美国加州大学圣地亚哥分校教授。1997年毕业于西安交通大学应用数学专业,获学士,2000毕业于中国科学院数学与系统科学研究院,获硕士,2006年毕业于加州大学圣伯克利分校,获博士。聂家旺教授主要从事多项式优化、张量计算、矩阵规划、非凸优化、凸代数几何、矩问题、大数据科学等领域的研究,是国际上该领域的著名学者。他曾获国际数学规划学会Tucker提名奖、美国国家科学基金杰出青年奖、美国工业与应用学会应用线性代数最佳论文奖。
报告题目三:A Constructive Approach to High-Dimensional Sparse Learning
报告时间:2019年12月25日(周三)上午 10:00
报告地点:西安交大财经校区教学楼806国际交流厅
报告摘要:
We develop a constructive approach to estimating sparse, high-dimensional linear regression models. The approach is a computational algorithm motivated from the KKT conditions for the $\ell_0$-penalized least squares solutions. It generates a sequence of solutions iteratively, based on support detection using primal and dual information and root finding. We refer to the algorithm as SDAR for brevity. Under a sparse Rieze condition on the design matrix and certain other conditions, we show that with high probability, the $\ell_2$ estimation error of the solution sequence decays exponentially to the minimax error bound in $O(\sqrt{J}\log(R))$ steps; and under a mutual coherence condition and certain other conditions, the $\ell_{\infty}$ estimation error decays to the optimal error bound in $O(\log(R))$ steps, where $J$ is the number of important predictors, $R$ is the relative magnitude of the nonzero target coefficients. Computational complexity analysis shows that the cost of SDAR is $O(np)$ per iteration. Moreover the oracle least squares estimator can be exactly recovered with high probability at the same cost if we know the sparsity level. We also consider an adaptive version of SDAR to make it more practical in applications. Numerical comparisons with Lasso, MCP and greedy methods demonstrate that SDAR is competitive with or outperforms them in accuracy and efficiency.
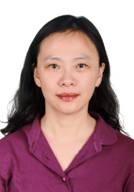
报告人简介:
刘妍岩,武汉大学数学与统计学院教授,博士生导师。2001年获武汉大学理学博士学位。主要研究方向为生存分析、半参数统计推断、高维数据统计分析等。主持完成国家自然科学基金以及教育部基金项目6项,正在主持国家自然科学基金面上项目一项,参加完成的成果“风险模型中的统计方法及相关理论与应用” 2013年湖北省自然科学奖三等奖(排名第一)。在统计学期刊Journal of Machine Learning Research, Biometrics, Biostatistics, Genetics,Lifetime Data Analysis等期刊发表SCI研究论文五十余篇。目前担任中国现场统计学会第十届理事会常务理事、中国教育统计协会常务理事、中国工业统计学会常务理事、中国数学会女数学家及西部数学发展工作委员会委员。
报告题目四:区块链技术原理及应用场景
报告时间:2019年12月26日(星期四)下午15:00-17:00
报告地点:西安交大财经校区教学楼614教室
报告摘要:
继大型机、个人电脑、互联网之后,区块链技术被认为是计算机模式的又一次颠覆性创新,很有可能在全球范围内引领一场技术革命和产业变革。区块链是一种分布式多节点“共识”实现技术,其本质上是分布式的数据库,包含交易、区块、链三要素。区块链具有分布式、不可篡改、交易透明、可追溯、智能合约、数据共享等特点。本报告将以公链为例,介绍区块链的定义,工作流程,并讲解非对称加密、挖矿、哈希、共识机制等区块链核心概念,以此理解其背后的技术原理。在理解其技术原理的基础上,探讨区块链适合做什么以及其存在的潜在风险,同时介绍区块链在金融科技领域的典型应用场景。
报告人简介:
李健,北京工业大学区块链研究中心主任,北京工业大学经济管理学院教授,博士生导师,教育部新世纪优秀人才,北京市长城学者,加拿大温莎大学访问学者。从事专业:管理科学与工程。研究方向:区块链技术与应用、金融科技、物流与供应链管理、应急管理。在Omega、IEEE Transactions on Systems, Man, and Cybernetics: Systems、International Journal of Production Economics、Decision Support Systems、《系统工程理论与实践》、《中国管理科学》、《管理科学学报》等期刊发表论文70余篇,由Springer出版英文专著2部。博士学位论文获2010年全国优秀博士论文提名奖。主持国家自然科学基金重点项目1项、面上项目2项、青年项目1项、教育部人文社科项目1项。兼任中国工业与应用数学会区块链专业委员会委员、中国指挥与控制学会安全防护与应急管理专业委员会总干事、中国系统工程学会监事会监事;中国科学院虚拟经济与数据科学研究中心虚拟商务研究室兼职研究员。其中专著Risk Management of Supply and Cash Flows in Supply Chains获得第七届高等学校科学研究优秀成果奖著作奖三等奖,参与的国家自科重大研发计划重点项目的相关成果获得四川省科学技术进步奖二等奖。
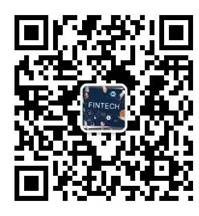
数字经济与管理周刊,欢迎扫码关注
数字经济与管理周刊依托北京工业大学区块链研究中心,关注国内外学术前沿,致力于聚焦最新的业界动态和研究热点,期待成为学子们心中的学术常青树。周刊关键词:数字经济、金融科技、智慧管理,满满干货!
经金学院
2019年12月17日